Tailoring Diffusion in the Melt to Optimise Solidification Microstructures, an Atomistic Approach.
With increased recycled content, the concentration of elements with low solubility and mobility, such as Mn and Fe, also increases, which can impair sheet formability and crashworthiness. Solidification sets the characteristic scale of the constituent particles spatial distribution. It is known that a finer and more uniform distribution of particles mitigates the loss of damage tolerance associated with a larger volume fraction thereof. Now the length scales generated during solidification result from a compromise between capillarity and diffusion. For a given cooling rate, slow diffusers demand more interfacial area between the growing dendrite and the liquid to partition between the two phases as dictated by equilibrium phase diagrams. The resulting dendritic morphology better distributes microsegregation than globular grains, which is what we’re looking for. The question is therefore: how is it possible to slow down the melt dynamics, that is, among other things, solute diffusivity in the melt?
Solute diffusion in the liquid metallic phase is a consequence of the atomic short range order (SRO) which prevails in the liquid phase. Constellium and the Quantum Engineering team of SIMaP have already collaborated in the past to improve the understanding of Al melts short range ordering. Icosahedral Short Range Order (ISRO) being the most compact local atomic arrangement is also the kind of order which most suppresses atom diffusivity: this is commonly called the “cage effect”. Now topological and chemical orderings (the same species may aggregate or different species may attract each other, depending on their electronic interactions), may concur or compete. This is what ab initio molecular dynamics (AIMD) allows to predict, and what molecular dynamics based on empirical potentials approximates.
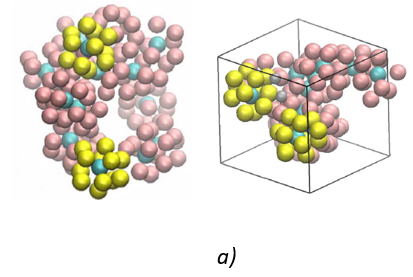
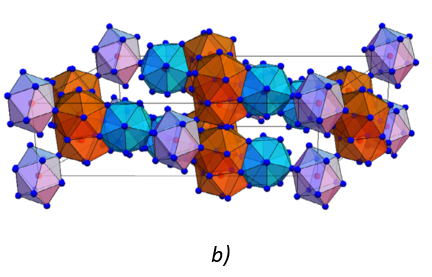
Cr additions promote the formation of a heterogeneous local ordering characterized by a strong five-fold symmetry (icosahedral short-range order (iSRO)) around Cr atoms. In the undercooled melt, AIMD evidences the extension of iSRO to icosahedral medium-range order (iMRO) (a) a most probable precursor of the 0-Al,Cr phase (b) which exhibits the pseudo-icosahedral symmetry of the Al4Cr icosahedral phase, i.e. 12 atoms located at the vertices of deformed icosahedra whose centres are occupied by a Cr atom. Although the size of the AIMD simulation is clearly too small to allow for the actual description of the peritectic reaction leading to the formation of e-Al,Cr, it evidences a spinodal decomposition of the liquid phase into two Dynamic Heterogeneities (DH), one with fast diffusion in the Al-richer DH and one with slow diffusion in the Cr-rich DH characterised by IMRO, which appears to be the liquid forerunner of that peritectic reaction. From: Medium Range Ordering in liquid Al-based alloys: towards a machine learning approach of solidification, Ph. Jarry and N. Jakse – ICASP6
AIMD demands huge computational resources. This is why this technique remains limited to the computation of small atom boxes, of the order of 500 atoms, with few different chemical species. In order to upscale this computational approach and to develop actual multiconstituent alloy design, the SIMaP team has started to use Artificial Intelligence through Machine Learning techniques to identify the parameters of empirical, implicit potentials able to mimic the behaviours predicted by AIMD. This is done in the framework of the Grenoble MIAI (Multidisciplinary Institute in Artificial intelligence) in collaboration with the LIG (Laboratoire d’Informatique de Grenoble).
Thanks to France Relance, the secondment of Philippe Jarry, senior scientist at Constellium Technology Centre and a solidification specialist, to serve as interface with, and guidance for, the research driven by Prof. Noel Jakse in SIMaP, could be funded. The postdoc of Alaa Fahs is also funded by this action, together with trainees. In the long term, this research avenue will gather sufficient data from AIMD to develop interatomic potentials able to provide reliable results at a low computational cost and at a larger scale, typically 106 to 107 atoms. This will pave the way to predicting not only SRO but Medium Range Order (MRO), thus filling the gap between present day AIMD and the nucleation scale and especially allowing for the computation of multiconstituent alloys. Concomitantly, Machine Learning approaches are being developed to identify atom arrangements by means of Topological Data Analysis.
Principal investigators
Philippe Jarry – C-TEC/SIMaP
Noël Jakse – SIMaP
Postdoc
Alaa Fahs